Future of Trading and Investing
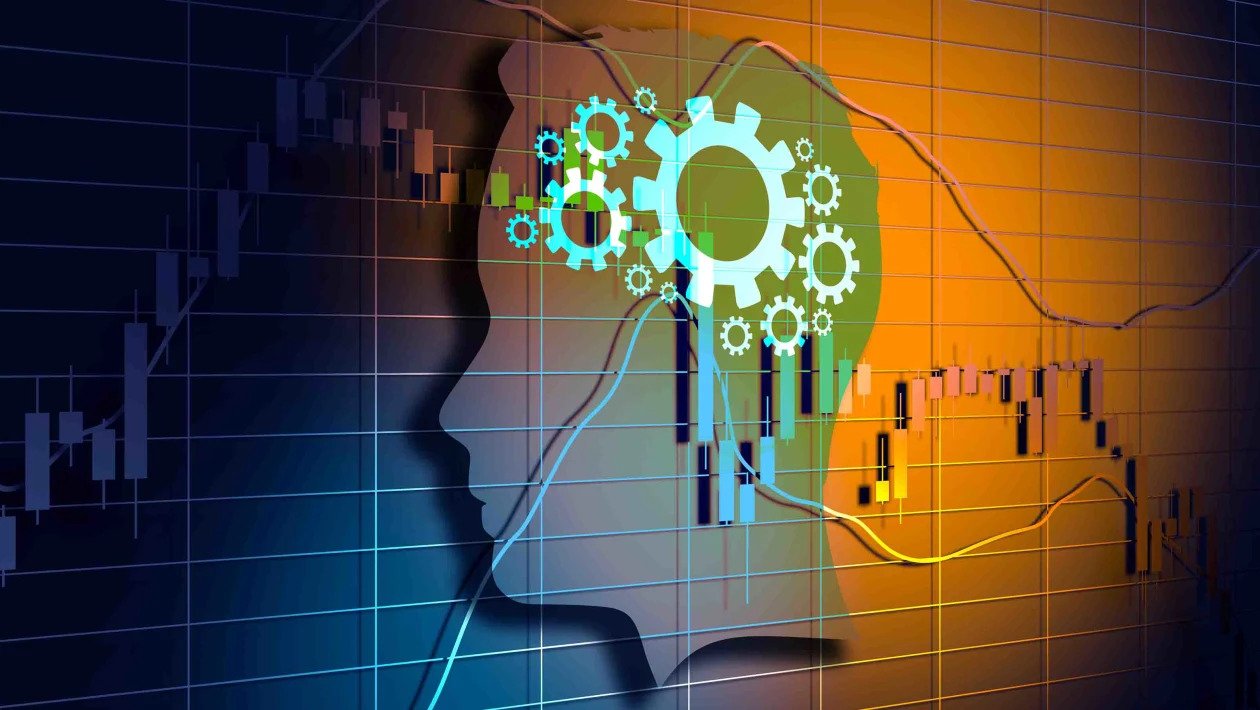
If data is now the most valuable commodity on Earth, how should it be used in the search for alpha? Find out more about the quantamental approach, which draws on unstructured data to create a blend of fundamental and quant investment research.
Today’s vast amount of data needs to be turned into structured content for investment research.
We are working on taming unstructured data, using AI, machine learning, natural language processing and textual data.
Blending fundamental and quantitative research is a powerful tool for finding alpha with unstructured data.
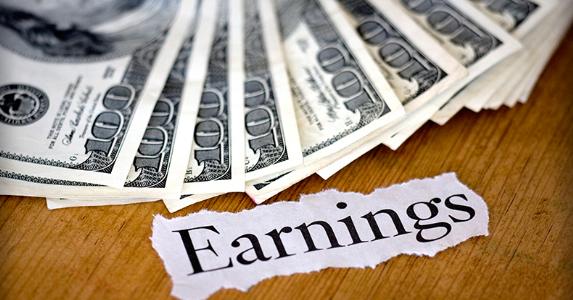
Predicting earnings quality
One way to find alpha is to enhance known anomalies and risk factors, or to create new factors based on discovered anomalies or less-traditional data sources. Regardless of these risk factors and exposures, blending them works better than any standalone solution.
Here’s an example: In addition to accruals data, free cash flow and operating returns seem to do a better job of predicting earnings quality.
When considering analyst revisions, instead of just looking at the EPS consensus change, look across the income statement to include EBITDA and revenue, and across multiple periods, not just the current quarter.
Another solution is to go beyond known anomalies and create new ones, such as a model of holdings that builds on ownership data using federal filings such as 13Fs, where institutional investors report portfolio holdings.
The model we created looks at what large investors added to their portfolios most recently and the underlying characteristics of those new purchases in order to identify their new idea generation screens.
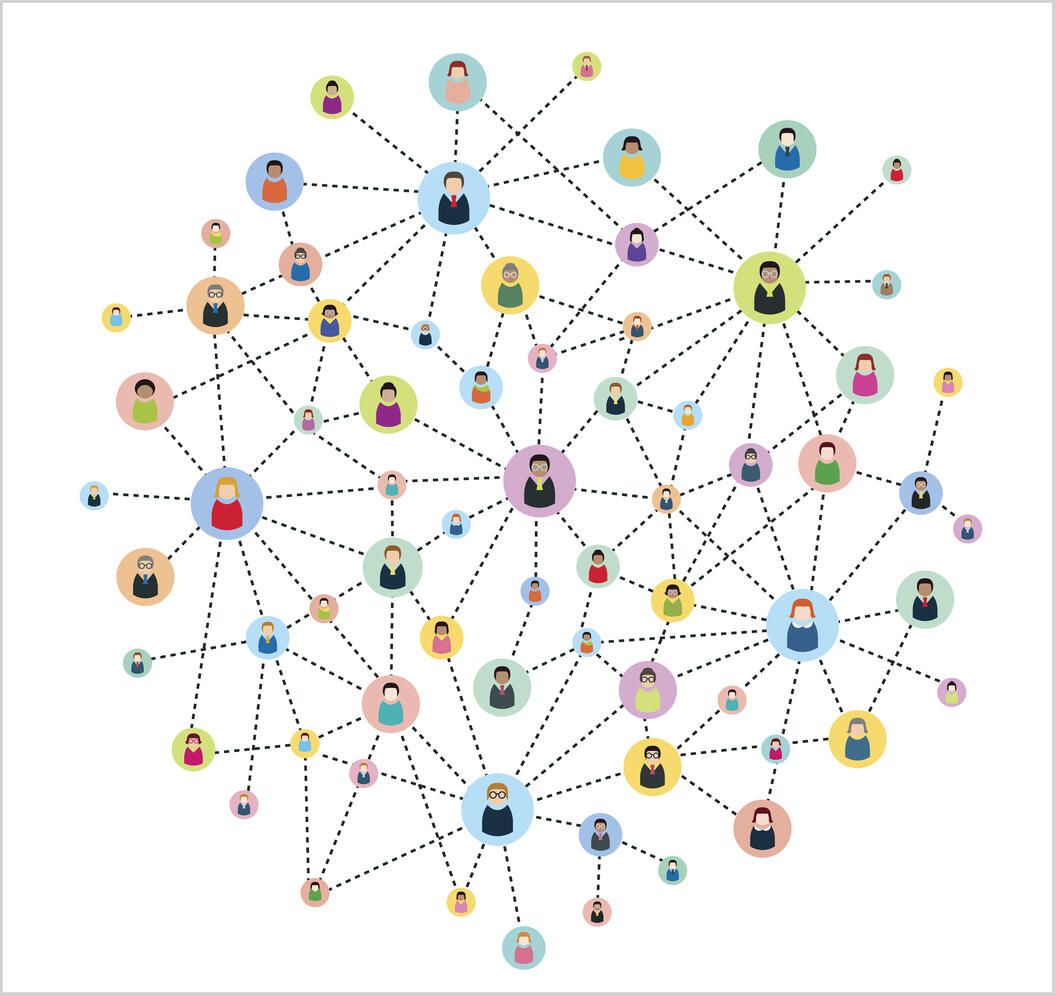
Using unstructured data
Many firms are now blending fundamental research analysis with quantitative research in a ‘quantamental’ approach.
However, it is easier to build quantitative models with well-structured data such as corporate financial statements. Today, 80 percent of data is unstructured and needs to be turned into structured content.
We are working on taming unstructured data, using AI, machine learning, natural language processing, and textual data. One example is using unstructured text and machine learning to assess a company’s credit risk and default probability.
Our model works with StreetEvents conference call transcripts, company filings, the Reuters news feed and selected broker research.
Each of those document types are dealt with differently, since the language used is different, depending on whether a lawyer, journalist or sell-side analyst created it.
Text from the document types is transformed into a profile where companies are ranked, with #1 being the riskiest and #100 least risky, based on the percentage probability of default over the next 12 months.
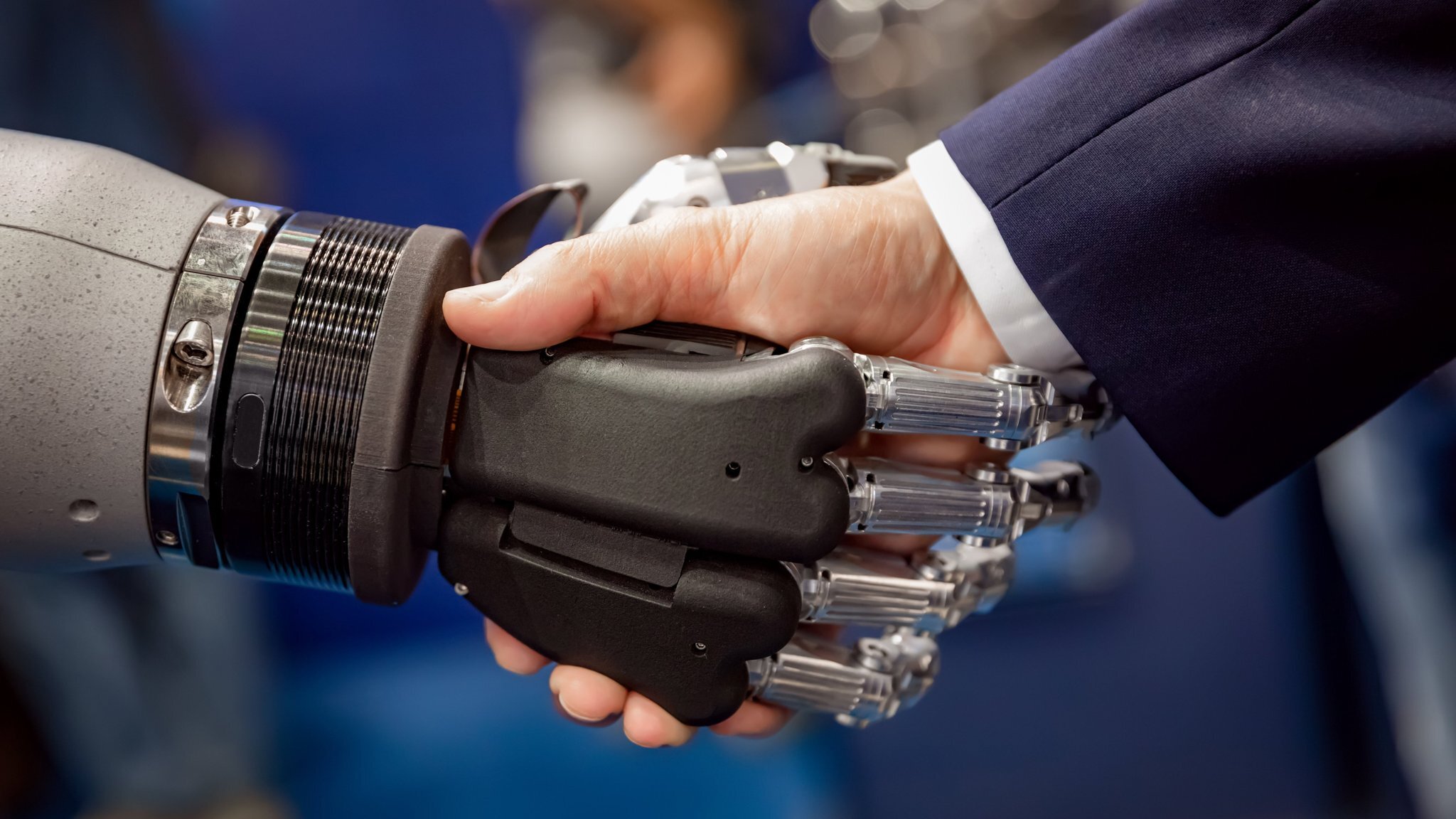
The quantamental approach
When the model was created in 2011, it analyzed “a bag of words,” assigning a value to such terms as “potential covenant violations.” Today, our research is looking at applying deep learning techniques to improve the credit risk models.
Large neural networks are being put to work on a bigger collection of language, instead of a bag of words, to see if the networks can create their own dictionaries of dangerous words and phrases.
Where is the future of data going? The quantamental area is growing, compared with a pure discretionary approach or entirely hardcore quant technique where a computer is taught to pick stocks. The key is a blend between smarter humans and smarter machines.